Podcaster: Dr Jacinta Delhaize and Dr Daniel Cunnama ; Guest: Dr Michelle Lochner
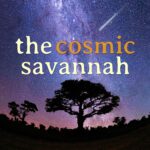
Title: Cosmic Savannah – Ep. 38: Rise of the machines
Link : www.thecosmicsavannah.com ; @cosmicsavannah (twitter, facebook & instagram) ; https://thecosmicsavannah.com/episode-38-rise-of-the-machines/
Description:
We are joined by Dr Michelle Lochner who is a senior lecturer at the University of the Western Cape, Cape Town, South Africa.
Michelle is developing new machine learning and artificial intelligence (AI) tools to analyse the massive astronomical datasets of next- generation telescopes.
Michelle talks to us about her work on detecting and classifying galaxies and transient events. She has also developed a code to spot weird astronomical anomalies – the so-called “unknown unknowns!”
She is preparing for the vast data influx of the Square Kilometre Array (www.skatelescope.org) and the optical Vera C. Rubin Observatory with its Legacy Survey of Space and Time (www.lsst.org)
Michelle is also the founder of the Supernova Foundation (www.supernovafoundation.org). This is a programme designed to inspire and support young women and gender minorities who are looking to pursue careers in Physics.
Bio: Dr Jacinta Delhaize and Dr Daniel Cunnama are astronomers based in Cape Town, South Africa. Jacinta is a Research Fellow at the University of Cape Town. She spends her time using huge radio telescopes to study gas and black holes in distant galaxies. Daniel is the Science Engagement Astronomer at the South African Astronomical Observatory. He likes to use large supercomputers to create simulations of galaxies. Both Jacinta and Daniel love to promote the incredible astronomy happening across the African continent.
Dr Michelle Lochner who is a senior lecturer at the University of the Western Cape, Cape Town, South Africa
Today’s sponsor: Big thanks to our Patreon supporters this month: Rob Leeson, David Bowes, Brett Duane, Benett Bolek, Mary Ann, Frank Frankovic, Michael Freedman, Kim Hay, Steven Emert, Frank Tippin, Rani Bush, Jako Danar, Joseph J. Biernat, Nik Whitehead, Michael W, Cherry Wood, Steve Nerlich, Steven Kluth, James K Wood, Katrina Ince, Phyllis Foster, Don Swartwout, Barbara Geier, Steven Jansen, Donald Immerwahr
Please consider sponsoring a day or two. Just click on the “Donate” button on the lower left side of this webpage, or contact us at signup@365daysofastronomy.org.
Please visit our Patreon page: https://www.patreon.com/365DaysOfAstronomy
or you can consider to sponsor a day of our podcast : https://cosmoquest.org/x/365daysofastronomy/product/sponsor-an-episode-of-365-days-of-astronomy/
Transcript:
[00:00:00] Dan: Welcome to The Cosmic Savannah with Dr. Daniel Cunnama
[00:00:09] Jacinta: and Dr. Jacinta Delhaize. Each episode, we’ll be giving you a behind-the-scenes look at world-class astronomy and astrophysics happening under African skies.
[00:00:18] Dan: Let us introduce you to the people involved, the technology we use, the exciting work we do, and the fascinating discoveries we make.
[00:00:26] Jacinta: Sit back and relax as we take you on a safari through the skies.
[00:00:33] Dan: Welcome to episode 38.
[00:00:35] Jacinta: Yeah. Hi everyone. Welcome back. And welcome to our new listeners.
[00:00:38] Dan: Yeah, today we will be speaking to Dr. Michelle Lochner, who will be talking to us about machine learning
[00:00:46] Jacinta: and artificial intelligence and how that can be used in astronomy.
[00:00:50] Dan: But before we get into that, you’ve been doing some podcasting outside of The Cosmic Savannah.
[00:00:55] Jacinta: Yeah, I have, but as a guest, several listeners here may have already heard it. I was the guest expert on the Jim Jefferies podcast “I Don’t Know About That”. Jim Jefferies is a comedian and every week he kind of has another expert guest about some different topic. And I did the latest episode on galaxies and it was a lot of fun and very different.
[00:01:21] Dan: Yeah, his style is a little different to ours. He’s a standup comedian. So I think he takes a completely different approach to podcasting.
[00:01:28] Jacinta: Did you hear it, Dan?
[00:01:29] Dan: I did. I gave it a listen. I thought it was great and you did really well.
[00:01:32] Jacinta: Thanks. His questions were very different. The conversation went in all different directions, but I loved it.
It was great.
[00:01:39] Dan: Yeah. If any of our listeners want to go check it up, excuse the hadadas over here, you can find it on any podcasting platforms. Also on YouTube where they have a video. And it’s called “I Don’t Know About That” with Jim Jefferies. And we should probably warn you that there is some strong language.
[00:01:57] Jacinta: Yeah, it’s a bit irreverent.
It’s not the same as our podcast. But actually talking about the Jim Jefferies podcast. I wanted to fact check myself a little bit. So maybe some of our listeners picked up a small mistake that I made, or maybe not. I don’t know. I said that most of the gold and silver on Earth were formed in supernova explosions, but actually that’s not true.
Most of it was formed in the collisions of two neutron stars. And I don’t think we’ve actually done an episode on that specifically, but we do have one coming up. Some of the gold and silver on Earth was formed in supernova, but most was in the collisions of pulsars. And I also said that it could also have been the collision of black holes, but thinking about that, that’s probably not true, is it Dan?
[00:02:46] Dan: No, that’s definitely not true.
[00:02:48] Jacinta: And the reason for that of course, is that nothing escapes from a black hole, not even light.
[00:02:53] Dan: Exactly. So yeah, in 2017 there was the first observed neutron star merger. And there we observed the remnants of that neutron star merger, and managed to work out that that’s where most of the gold and silver, along with many other elements actually, is formed.
[00:03:11] Jacinta: Yeah. And I’ve actually done a long blog post about that. So I really should have known this. You can go to my website, jacintadelhaize.com and it’s on my blog. And it’s the first blog post that I did. And you can read all about that if you’re interested. So there we go. It’s important to fact check even ourselves.
And you have also been doing something quite exciting recently, Dan? Yeah.
[00:03:32] Dan: I’ve been engaging in some construction myself. I’ve probably mentioned it before. We’re building a new visitor center here in Cape Town, which will hopefully open later this year. And. Yeah. So this morning I was Bob the Builder. Had my hard hat on and my safety boots and we lifted a new telescope, a solar telescope onto the visitors’ center roof, which was super exciting.
Got to play with the crane, although I didn’t drive it. And yeah, super fun.
[00:04:00] Jacinta: Great, and what are you installing?
[00:04:02] Dan: So it’s called a heliostat and it has a telescope which will observe the Sun through the day and project an image of the Sun into the room below. So we will be able to see a live view of the Sun. And if there’s sun spots or any interesting things on the Sun to see, then we’ll be able to see those, but it’ll also have a spectrograph on it.
And a spectrograph essentially splits up the sun’s light into all of its constitute wavelengths. So we’ll be able to have a spectrum of the Sun a live spectrum of the Sun, which we’ll also be able to observe.
[00:04:35] Jacinta: Awesome. Did you just say that you got to drive it yourself?
[00:04:38] Dan: No, I didn’t get to drive myself.
[00:04:40] Jacinta: Oh, because that would be fun.
[00:04:43] Dan: That would have been a boyhood dream. Yeah.
[00:04:46] Jacinta: But maybe not breaking the telescope, that may not have been so good. Cool. All right. Well, shall we talk about today’s episode now that we are, how many minutes in?
[00:04:56] Dan: I think we should.
[00:04:57] Jacinta: Okay, great. Yes. As you said today, we are talking to senior lecturer from the University of the Western Cape, Dr. Michelle Lochner. And she’s going to be telling us all about machine learning and AI and neural networks and all of these really amazing things, which I’ve heard very little about, I didn’t know much of this at all. And so it was very interesting to talk to Michelle.
[00:05:22] Dan: Absolutely. I think that, you know, not a lot of people know about this, and it’s a very new field in astronomy and very exciting because it’s opening up a whole new avenue of astronomy in terms of the dealing with the big data that we have and trying to work through that at a level that humans can’t actually keep up with.
[00:05:41] Jacinta: So Michelle explains all about that and her work on the MeerKAT telescope and also the Vera C. Rubin telescope, which is performing the LSST. The, help me out here Dan, Large Synoptic Survey telescope?
[00:05:54] Dan: Legacy
[00:05:55] Jacinta: Legacy. Okay. What is it?
[00:06:00] Dan: Legacy Survey Telescope.
Maybe Synoptic.
[00:06:07] Jacinta: Okay. We’ll Google that in the meantime. No, Michelle will tell us, Michelle will tell us. Without further ado. Let’s hear from Michelle.
With us today we have Dr. Michelle Lochner, who is a senior lecturer at the University of the Western Cape in South Africa. Welcome Michelle.
[00:06:27] Michelle: Hi Jacinta. How you doing? Good thanks.
[00:06:30] Jacinta: We’ve got Dan here as well. Welcome to The Cosmic Savannah.
[00:06:32] Michelle: Hey Dan.
[00:06:33] Jacinta: Thanks for joining us today, Michelle. Just to start off, can you tell our listeners a little bit about yourself, who you are etc.
[00:06:40] Michelle: Sure. So I’m Michelle Lochner. I’m a senior lecturer at the University of the Western Cape, but I also have a staff scientist position at the South African Radio Astronomy Observatory, which I’m sure by now everybody knows about because of the exciting MeerKAT and SKA telescopes. And I’m interested in a lot of different things in astronomy and cosmology, but in particular applying machine learning and other cool data science techniques to try and handle the massive amounts of data coming from our amazing modern telescopes.
All right. So first of all, what is machine learning?
Yeah, sorry, maybe not. Everybody’s heard of machine learning, but I’m sure everybody has heard of artificial intelligence, or AI. That’s definitely been part of our scifi viewing for many years. So artificial intelligence is really any computer program that is making decisions.
So you find it on your phones, on airplanes, even in your washing machine. But machine learning is a particular type of artificial intelligence that’s become really, really important in recent years. And the idea behind machine learning is that it can learn to do things without being explicitly programmed.
So it’s an algorithm that can teach itself by looking at data. And this has become really important for everything. There are many machine learning algorithms running on your phone, running in your email, maybe recommending good music to you. But it’s becoming more and more important in astronomy as well to try and handle in automated ways, the huge volume of data that we’ve got coming in. That up until now, it’s been small enough for humans to be able to do everything. We’re now starting to have to rely on the machines a lot more.
[00:08:26] Dan: How do you set up a machine to learn about astronomy though?
[00:08:30] Michelle: That’s a great question. So you always have to start with data, right?
So you’ve got to start with your data and you’ve got to start with a question. So a great example in the ordinary world is, say you want to be able to tell the difference between cats and dogs. And this is a very important question that needs to be solved. So you would have to run a machine learning algorithm, which is designed to figure out the difference between cats and dogs in an automated way.
And you’ve got to start with a dataset, a training, set of known examples of cats and dogs. There are many different algorithms that you can choose. For instance, one called neural networks, which are very popular, which is based on how we think the human brain works. And is made up of these connected neurons that you can then train with your training set of known examples of cats and dogs.
And once you have a trained neural network, you can apply it to new data to say, Hey, is that a cat or is that a dog? And these are the algorithms that Google for instance, is running all the time. If you’ve ever done a Google image search, it’s running a neural network in the background.
We do this pretty similarly in astronomy. We may, for example, have a sample of spiral galaxies and elliptical galaxies. And years ago people would do this manually look through the data and say, yes, that looks like a spiral. Or yes, it looks like an elliptical. But we now regularly have datasets with millions of objects. And it’s just, there’s just no way a human can go through all of them.
So we would create a training set of known spirals and ellipticals. Train for instance, a neural network algorithm to be able to tell the difference and then apply it to larger datasets, that we can then do some science with. That’s a type of machine learning called supervised machine learning. That’s very popular. But I actually work in a different branch of machine learning mostly, called unsupervised learning.
[00:10:28] Jacinta: Okay. I have so many questions. I don’t know where to start. Okay. So we’re going to find out about unsupervised learning and in a second, but first of all, you said that we start with huge amounts of data that we’ve got huge amounts of data at the moment. So we can’t look through all of it by eye how much data do we have?
[00:10:44] Michelle: It depends on the dataset, but already the MeerKAT telescope, which is the precursor to the SKA telescope, which will be sort of the, the biggest telescope on Earth. MeerKAT’s already producing terabytes of processed data. So unprocessed data is much larger. But these are even final images, radio images, and they’re in the terabytes. It’s already too big to download onto your laptop.
And each image might contain thousands of radio galaxies. Then you’ve also got huge optical surveys being done with a variety of telescopes around the world. That’s easily producing a catalog of a billion galaxies. And you know, now you have to try to search through all of these.
Here’s another number to overwhelm you. The Vera C Rubin observatory, which I’m sure we’ll talk about a little bit later, it’s a telescope being built in Chile. Here’s a cool number. It’s such a powerful and sensitive telescope that it will detect 10 million transients every night. So 10 million times a night, something on the sky will change and this telescope will detect it.
And some fraction of those will be interesting, cool objects that we’ve never seen before that we want to follow up. But that’s 10 million, every single night. That we somehow have to process and do some science with. So the numbers are getting just more and more ridiculous than more telescopes we build.
[00:12:08] Jacinta: That’s staggering! 10 million per night. What??
[00:12:11] Michelle: Yeah.
[00:12:15] Dan: We should probably just clarify what a transient is too. I mean, so we’ve talked about transients once before, so these are things that essentially go bump in the night. And now we hear that there’s 10 million of them, it’s a lot of bumps. So there could be things like supernova, right? Exploding stars or those sorts of things, but what else are you detecting when you’re detecting transients?
[00:12:36] Michelle: Yeah, that’s right. So I mean, about half of those 10 million things are not going to be astrophysical at all. They will be cosmic rays or airplanes, or of course, satellites. Lots of them will be satellites.
[00:12:50] Jacinta: Ah,the satellite constellations.
[00:12:52] Michelle: Yeah, unfortunately.
[00:12:54] Dan: Starlink.
[00:12:54] Michelle: Yeah. Starlink is a real problem for optical telescopes. But you know, it’s still about half of those are going to be actually interesting things.
So supernova is one that you mentioned, which are massive explosions of stars .Within our galaxy there’ll be things like variable stars. So these are ordinary stars that pulsate and change. There will be active galactic nuclei. So these are, I’ve heard them called “galaxies behaving badly”.
[00:13:24] Jacinta: Where did you hear that?
[00:13:27] Michelle: I’m pretty sure it was Prof Wilcotts who said that. It’s just stuck in my mind. Galaxies behaving badly. So supermassive black holes inside galaxies, devouring things and spitting out lots of radiation. Of course. There’s the rare kilonovae that we’re hoping to find a few more of that, we’ve only ever detected one of.
[00:13:47] Jacinta: These are the most powerful of the supernova, right?
[00:13:50] Michelle: Well, these are the results of a binary neutron star merger. And if you remember the gravitational wave events of a few years ago, that made such a stir in astronomy, that’s the one and only kilonova we’ve detected. So there a gravitational wave was detected and then telescopes followed up and then found the kilonova, right.
But it’s possible that in amongst these 10 million transients every night, a couple of them could be kilonova. So that’s a real needle in a haystack problem. And of course there’s all kinds of things that we can’t even predict now. I mean, maybe there will be new types of transients that we haven’t discovered up until now.
These are the things that I’m really excited about.
[00:14:35] Jacinta: Okay. So now we’re talking about transients, but let’s get back to the machine learning. Okay. So there’s going to be all of these things that this telescope, the Vera C. Rubin telescope and, you know, MeerKAT and the SKA. They’re all going to be detecting these strange things.
And so it’s just impossible for humans to go through this and find them all themselves by eye. So we need computers to do this now. So you’re talking about machine learning, like artificial intelligence, and one type of that you said is called neural networks. So can we just go back to that? I didn’t quite understand.
So you said it’s kind of like the human brain now is, I don’t know anything about this. So are you able to describe that in a way that I might be able to understand what a neural network is?
[00:15:15] Michelle: Sure. Okay. Let me try. So what is a machine learning algorithm do? It’s often referred to as a black box. It’s a thing that takes inputs, does some stuff and produces outputs.
Okay. That’s one way of thinking of a machine learning algorithm.
[00:15:35] Jacinta: I’m still with you now.
[00:15:37] Michelle: Good. In the example of telling the difference between cats and dogs, the input would be an image. So some kind of picture and the output would be cat or dog. Right. So it’s the prediction of what should this label be for this image?
Okay. So the question is what’s all the bits in the middle? What’s the stuff doing that goes from an image to cat or dog? The bit in the middle is the algorithm itself. And there are many different ones. You could think of it as this complicated mathematical model and there’s many different ways of building them.
So a neural network will take an image and pass it through what’s called a layer of neurons. So these are like neurons in the brain and there can be many layers of neurons that are connected in some particular way.
[00:16:31] Jacinta: Okay. But when we say neuron, sorry for interrupting you there, but it’s not like an actual neuron in our brain.
It’s some piece of code, right?
[00:16:38] Michelle: It’s a piece of code. Yeah.
[00:16:39] Jacinta: Okay.
[00:16:40] Michelle: Yeah. It’s a piece of code.
[00:16:41] Jacinta: There’s like little chunks of pieces of code that it’s passing through.
[00:16:43] Michelle: That’s right. That’s right. So you can think of it as these pieces of code that we use mathematics to connect to each other. The actual training part learns how important each bit of code is in being able to transform from the image to the label.
[00:17:01] Jacinta: Okay. So it’s deciding the importance.
[00:17:03] Michelle: Yeah, exactly.
[00:17:04] Jacinta: Of each of these bits of information.
[00:17:05] Michelle: Yeah.
[00:17:06] Dan: Basically for the cat and dog example, right. The neural network is going to learn, cats have pointy ears, dogs have sort of soft or fluffy ears. And therefore the ear neuron is an important one. Is that about right?
[00:17:22] Michelle: Yeah. Yeah. You can, you can think of it that way. Yeah. It ends up…
[00:17:27] Jacinta: it’s hashtag complicated.
[00:17:30] Michelle: It is complicated. It ends up being along those lines. Yeah. You could say that some parts of the neural network will be sensitive to the, you know, the color or the overall shape of the animal. Some parts will be sensitive to the length of the tail and the size of the ears.
So it does end up working out kind of like that. Yes.
[00:17:51] Jacinta: Okay. But we’re not actually looking at pictures of cats and dogs. We’re looking at pictures of galaxies, right? Or that’s what your work does. Maybe can you tell us more about that?
[00:18:01] Michelle: What I’m interested in is a different branch of machine learning, where we don’t know what we’re looking for.
The most common types of machine learning that people work on is like the cats and dogs example, where we have some training set. We have some known thing that we’re looking for and we can train a neural network, for example, to look for that thing. I am more interested in finding the unknown unknowns. So the things that we didn’t know we should have been looking for, and that’s quite a bit harder because of course you don’t have your training data or you don’t have any known examples by definition.
And so what I do is I might take a dataset of galaxies such as from the MeerKAT telescopes. So I’ve all these beautiful radio galaxies. And I want to run a version of machine learning called anomaly detection. So looking for all the rare galaxies in this dataset. So what I have to do is find… This actually gets even more complicated than neural networks.
[00:19:11] Jacinta: What?? What are we going into now?
[00:19:15] Dan: I can imagine.
[00:19:17] Michelle: Let me see if I can describe this. So this actually works quite differently to the neural networks. What I do is I find a way to describe the shapes of galaxies, a kind of mathematical way. And I do this by asking the question, how similar is this galaxy to an ellipse?
Because a lot of the time ordinary boring galaxies that aren’t doing anything interesting, just look like ellipses. Whereas things like merging galaxies, for instance, or just weirdly shaped galaxies, interacting galaxies, don’t look like ellipses. They look like they have strange shapes. So I do something called feature extraction, which is a way of simplifying your data down to a simple set of numbers. And in this case, my numbers describe the shapes of galaxies using these ellipses. Okay. Are you with me?
[00:20:18] Jacinta: Yes.
[00:20:19] Michelle: Okay.
[00:20:19] Jacinta: Dan, maybe you can describe what Michelle just said.
[00:20:26] Dan: I mean, I think the thing is, right. So you’re looking at something like a galaxy and you don’t really know how you want to classify it. And you don’t know that they come in spirals or ellipses or whatever. So you compare it to a square. Nothing really looks like a square. You compare it to circle. Some of them look a bit circular.
You compare it to a few other things. The computer builds up an understanding of how these things look on its own. And then it looks deeper at the various galaxies and sorts them into what it thinks are a good classification system. So maybe the computer won’t come up with the same classification system as we would in terms of spirals and ellipses. And maybe it’ll have some new idea. And I think that’s where it gets really interesting because you start to see patterns and information, which the human eye or human brain maybe wouldn’t have seen.
[00:21:23] Michelle: Yeah, that’s a great summary.
Yeah!
[00:21:27] Jacinta: Well done!
[00:21:27] Michelle: Yeah.
[00:21:29] Dan: Yes, yes. Now I want to take it to the next step. So these transients, right?
We’re getting 10 million transients a night. Now, you know what a Starlink satellite looks like when it goes over. Presumably you know what an airplane looks like. Although depending on its path, it might be a bit different. And you know what a supernova is. So, you’ve got a trained dataset already for these, but then do you run a concurrent unsupervised algorithm on that to try and find things which we didn’t know existed?
[00:22:00] Michelle: Yeah, that’s good. So, the supervised algorithms will always try and give you an answer. They’ll always tell you the closest thing that this thing looks like from its training set, even if it’s completely wrong. So supervised algorithms are really designed to classify no matter what, even if it does quite badly.
So you do have to run a separate anomaly detection to try to find things that it’s never seen before. The classifiers just aren’t designed that way. It’s a completely different type of algorithm you have to run.
[00:22:29] Jacinta: So then what’s unsupervised learning?
[00:22:32] Michelle: It’s not even a great name, really. Supervised learning simply means you have a training set. So you know exactly what you’re looking for. So you can use that training set to train an algorithm to, for instance, classify different types of galaxies. Unsupervised learning means you don’t have a training set. You don’t know what you’re looking for. And so, the two kind of branches of unsupervised learning are basically exactly what Dan, hit on, is something called clustering. Looking for things that even though the algorithm doesn’t know what they are, it knows they look similar and indeed, sometimes they come up with different groupings to what we would normally do. And then the other thing you can do is what I’ve been working on, which is anomaly detection. So in the example where we are fitting shapes to the galaxies, I’m looking for ones that are a bad fit. Right. They don’t look much like the shapes of most of the galaxies in the dataset. So really looking for any kind of outliers or abnormal galaxies.
[00:23:44] Jacinta: Okay. So that makes sense. Now, can you give us some examples of what kind of anomalies you’re finding or, you know, what kind of clusters you’re finding?
[00:23:53] Michelle: Yeah. Probably the type of anomaly my algorithm is so far been most sensitive to is, in the optical anyway, merging galaxies. So these are two galaxies that are literally merging, joining to become one or just galaxies that are interacting. So sometimes, you know, you see these big streams of stars and gas being stripped from one galaxy to another, tidal streams. So really, anything that’s got an unusual shape. I’ve picked up a couple of strong lenses for instance. Yeah. So basically anything with unusual shapes. In the radio…so radio galaxies are really fun. Especially the anomalous ones.
[00:24:38] Jacinta: I agree.
[00:24:40] Michelle: I’ve been mostly picking up any kind of radio galaxy that’s interacting. So usually interacting with the intergalactic medium. The kind of standard radio galaxy has these big jets that come out of the supermassive black hole. And if these jets are interacting in some way with the intergalactic medium, it gives them interesting shapes. So you see these bent tail galaxies and just things that have strange morphologies. Those are the types of things that I’ve been mostly sensitive to.
[00:25:13] Dan: So you’re working on MeerKAT, right? And you are detecting these anomalies and galaxies. Now I don’t want to keep going back to the Vera Rubin Observatory, although I do love a good transient.
[00:25:26] Michelle: Who doesn’t?
[00:25:28] Dan: It’s quite obvious why we want to identify anomalies, because you want to find something that went bump in the night that you don’t know what it is because that’s something potentially interesting. What do you learn from detecting anomalous galaxies or some sort of galaxy which doesn’t fit the standard?
[00:25:48] Michelle: Ah, that’s a great question.
[00:25:51] Jacinta: I was going to ask the same Dan, by the way!
[00:25:52] Dan: Of course you were!
[00:25:58] Jacinta: Actually I was, and I wanted to say like, Michelle, what do you think the scientific benefit of this is? So all of that in one question.
[00:26:05] Michelle: Right? I mean, that’s a great question. In science, we always try to break whatever is the status quo, right? So whatever is the current model of the universe, we’re looking for things that break it, right?
So the idea of finding unusual galaxies is that it can help improve our understanding of all types of things, but basically how these galaxies evolve and how they work. So I’ll give you an example. MeerKAT’s a great example because MeerKAT is really more sensitive than any telescope that’s come before it. So it is starting to find radio galaxies. That really don’t quite look like anything we’ve seen before. We’re starting to see much finer detail than we’ve ever seen before. And so it’s been able to answer some questions of how these galaxies actually form you know, how they’re interacting with each other. Just understanding more about galaxy evolution.
And my thinking is that when we have billions of galaxies in a dataset, it’s very likely that some of these interesting things will be missed. And we really are looking for things that either challenge our current models of how we understand galaxy evolution, or help answer questions that we haven’t been able to answer until now, because we just didn’t have sensitive enough data.
I’m never looking to answer any particular science question, because that would mean I know what I’m looking for, right? But once we find something weird, the next step is to go, okay, well, what is this? And what does it mean? What do we learn about the universe from this weird and unusual object?
[00:27:52] Dan: So the question which I guess a lot of people would ask is, are you not putting astronomers out of a job?
[00:27:59] Michelle: That’s a great question. Okay. So my work is all about when we have these datasets of billions of objects and we know it’s not possible for a human to search through all of these datasets to find interesting objects. What do we do?
How can we automate scientific discovery? If we can automate scientific discovery. Yes, it’s true. Surely we’re putting all astronomers out of a job. What are PhD students going to do with their degrees? Well, actually, conversely, I think these algorithms are really important to enable scientists to do their jobs.
And in fact, the more I work in machine learning, the more I realize how important it is to have a human involved in making the final decisions. No machine learning algorithm is a hundred percent perfect. And every machine learning algorithm really actually needs improvement. So a lot of the time when people apply machine learning, they apply it to some known dataset and they say, oh, look, I got an accuracy of 97% and then that’s done.
But how do you use them in the real world? How do you use them on real scientific datasets? It’s called human in the loop learning. So having the human involved to improve the machine learning algorithm is really critical. So, what I’ve been working on is a publicly available software called Astronomaly.
I’m very proud of the name
[00:29:32] Jacinta: I love the name because it’s astronomy anomally. Astronomaly!
[00:29:38] Michelle: It turns out to be really hard to say, but anyway.
[00:29:40] Dan: Thanks for explaining it, Jacinta.
[00:29:41] Michelle: So the idea behind Astranomally is that if you’ve ever watched Netflix or whatever streaming service and it says. Oh, we think you’d like to watch this thing next. It’s usually completely wrong, but you know that there are these things called recommendation engines, which are being used more and more like for online shopping or for music streaming, et cetera.
And so the idea behind Astronomally is building a recommendation engine for, scientific. Discovery specifically in astronomical datasets.
[00:30:18] Jacinta: Now, the important question. If these networks, these learning algorithms are unsupervised and while we’re busy, not micromanaging them, are they going to rise up and are the robots are going to come and kill us?
[00:30:32] Michelle: Yes, I think it’s the inevitable end the human race.
To be honest, for all the incredible things that machine learning can do it really can do some amazing things. These algorithms are quite specific and quite specialized and they are just not that smart, at least not yet. I’m personally, right now, not very worried about the robots. I, you know, I have about my toaster rising up against me. It will come eventually. I mean, it’s, it’s inevitable if you can, if you can build general artificial intelligence, but I personally don’t think we’re very close to that.
[00:31:14] Jacinta: Okay. So no Terminator just yet.
[00:31:17] Dan: So we talked a little bit about the transients already, and this is part of the Vera C. Rubin Observatory, which is coming soon. You’re involved in that quite heavily. Aren’t you?
[00:31:26] Michelle: Thats right. The Vera C. Rubin Observatory is a really exciting project. It’s a telescope being built in Chile as mostly a US led project, but there’s a lot of international involvement as well. And South Africa got involved a few years ago and now it’s getting even more involved, which is really exciting.
So this telescope will be just, it’s almost like the SKA, but for optical. It’s going to do this incredible survey of the entire Southern sky. And South Africa bought in or got involved a few years ago and I was announced as one of the three South African principal investigators.
[00:32:04] Jacinta: Congratulations, by the way.
[00:32:05] Michelle: Thank you. Which means that I get access to the data immediately, as soon as it comes off the telescope and, you know, get to work on it. Get to be involved basically. What’s really exciting is that we’re increasing the number of PI’s available. So more South African scientists are gonna get involved.
And the cool thing about the Rubin observatory is that although the data is not available immediately, it is made public after some time. And there’s a lot of work being done in preparing these incredible platforms for the general public to actually have access to the data and work with it. So I’m working on setting up anomaly detection on Rubin data. And my hope is that citizens all over the world can get involved in this citizen science project and maybe even make amazing scientific discoveries in this public dataset. So that’s what I’m really excited about for this project.
[00:33:04] Jacinta: That’s so awesome. And when you say, you are the PI, are you the PI of a particular project or is this a general thing?
[00:33:11] Michelle: Obviously I wrote a proposal for the type of work that I wanted to do, which was all around machine learning, specifically, working on both MeerKAT and Rubin data together. So multi wavelength stuff. But yeah, so even though it’s a general proposal around a particular area. It’s not the same as a PI of a specific project.
So I lead the team. I have students and post-docs on my team working in this area.
[00:33:37] Jacinta: Oh, okay. So like the telescope just takes the data of the whole sky every night. And then you’re the PI of a particular research project.
Is that right?
[00:33:46] Michelle: That’s right. It’s quite different, for instance, with MeerKAT, you would write a research proposal and then you get your data to work on your project, for whatever.
[00:33:56] Jacinta: Yeah. You kind of request time to look at a particular part of the sky.
[00:34:00] Michelle: Yeah, exactly. Whereas the Rubin Observatory’s undertaking this thing called the LSST. So the Legacy Survey of Space and Time, and it’s a 10 year survey. So basically they’re just doing this massive single survey. And then everybody gets access to the data to do whatever science they want.
So I’m very involved in the cosmology side. For instance, people are doing transients, people are doing solar system studies, galactic studies. It’s quite ambitious that you have this single massive survey out of which tons of science should come.
[00:34:34] Jacinta: And the telescope is enormous as well, right?
[00:34:37] Michelle: Yeah. So it’s a, it’s a 10 meter class telescope, but what’s, what’s incredible about it. Really. It’s got very clever optics and it’s also got the biggest camera in the world on this telescope. It’s wow. It’s like a four gigapixel camera. It’s just crazy. The physical camera. I think it’s, it’s like a two meter diameter camera.
I can’t remember the exact number, but it’s something like that.
[00:35:03] Jacinta: Awesome.
[00:35:03] Michelle: It’s really massive.
[00:35:05] Jacinta: wThank you so much, Michelle. And just before we go, I wanted to ask you about one more thing. Not only are you an amazing scientist and a wonderful role model, you also take a lot of care for the wellbeing of the community and you run something called the Supernova Foundation. Would you like to tell us a little bit about that?
[00:35:24] Michelle: Yeah. So the supernova foundation is a mentoring and network program for women and gender minorities in physics. So it’s a program I set up a few years ago and it’s growing. We have about 400 members now from all over the world. Over 50 countries I think are represented.
So the idea is that we try to connect senior women physicists. Women and gender minorities with students from all over the world who are likely to be in environments, which are male dominated. So they don’t necessarily have role models in their immediate environment. It’s a virtual platform, which has been great for during the pandemic, especially, yeah, basically mentors and mentees meet up usually around once a month for mentoring and we have webinars and we basically have just tried to kind of build a community.
And it’s been a really amazing, really positive experience. I think we’ve had some great impact on quite a few people around the world.
[00:36:24] Jacinta: That’s awesome. So if wthere are some women or people of gender minorities listening to this, who would like to get involved, participate in this either as a mentor or mentee, how can they do that?
[00:36:36] Michelle: Sure. Yeah, you can just go ahead on the website, supernovafoundation.org, there’s a signup page. So if you are interested. We would very much welcome here.
[00:36:48] Dan: Awesome. Thanks. I mean, it’s excellent work and we will definitely post those links on our website too. Before we go. Is there any final message you’d like to send to our listeners?
[00:36:59] Michelle: What can I say? Well, machine learning is something that’s in everybody’s lives in your phone, in your computer, in everything you do. Like many things, it can be used for great things and it can be used for some not so nice things. But I hope after listening to this, you’ve seen how excited I am about using machine learning and astronomy, where I think it’s being put to great use, getting the best science out of amazing telescopes, like MeerKAT. So I hope your listeners are as excited as I am about it.
[00:37:35] Jacinta: Certainly after this. Thank you, Michelle. Thank you for your passion and thank you for your work.
[00:37:38] Michelle: Great. Thanks so much Jacinta. Thanks Dan.
[00:37:41] Dan: Thanks Michelle.
[00:37:48] Jacinta: So the Legacy Survey of Space and Time, LSST. We weren’t even close Dan.
[00:37:55] Dan: Well, we learned something today. I think I learned a few things today, actually. Yeah. Very interesting conversation with Michelle.
[00:38:02] Jacinta: This machine learning thing is really cool and I’m really excited about it. So if I can talk about radio galaxies for a second now, Dan, is that ok?
[00:38:10] Dan: Sure.
[00:38:12] Jacinta: So our regular listeners will know that I work a lot on radio galaxies and radio surveys. And these are wgalaxies that have supermassive black holes in the center releasing huge amounts of radio light. And when we look through our data, we can just see lots of them, but they have quite complicated shapes. And one blob over here may be associated with another blob over here in the same system, but they kind of look separated on the sky and what Michelle’s algorithm does partly or other algorithms like that, it actually find those blobs and associate it with the same thing. And this takes hours and hours and hours and hours of our time. Certainly I’ve spent a lot of time myself trying to figure these things out and to have a computer program that will do it for you is going to be really kind of game-changing is the word.
[00:39:02] Dan: Ground breaking.
[00:39:03] Jacinta: Yeah. Yeah, exactly. It’s the word I’m looking for. And I love that Michelle was saying was kind of calling this automated scientific discoveries. And she said, you know, it’s not the computers that are making the discoveries and writing the papers. It’s freeing up our time to actually do the science.
You know, we don’t need to go and identify these objects. The computer identifies them for us. And then we can follow up and do the science and understand what they are and write the papers. So this is really exciting for me.
[00:39:29] Dan: Yeah, it’s super exciting. I think one of the other things that’s worth noting is that when you’re doing this sort of work, like you’re trying to identify radio galaxies or transients or whatever, we don’t always have perfect data.
So, you wknow we don’t get a perfect light curve or a perfect observation every single time or a perfect map of a galaxy. We always get like parts of it and sometimes different parts of it. These machine learning algorithms will be better at sort of piecing together the full picture based on an incomplete puzzle, which I think is very cool.
And I think that’s like you said something which is very difficult at the moment to try and piece together these observations yourself. So having some sort of algorithm which can do this efficiently and accurately or more accurately, it really is as you say, going to be a game changer. Yeah.
[00:40:21] Jacinta: And the fact that Michelle is actually applying it mostly to the unknown unknowns. That is so cool. Like whenever you build a new, big telescope like MeerKAT or the SKA or the Vera C. Rubin Observatory, you have to design it with science goals in mind because you have to make sure that it is going to discover the things that you are hoping that it will discover or be good enough to do the science that you want it to do.
But it’s really hard to design a telescope that is good for discovering something that you don’t know exists. These are the unknown unknowns. And so I love that Michelle is taking the data that we already have and finding, looking for unknown unknowns. And this is where the really groundbreaking stuff is in like finding things that, you know, our current algorithms or our current, even our own brains may miss, because we aren’t looking for it.
Michelle’s going to find those things. So maybe some really exciting things could come out of that.
[00:41:20] Dan: Yeah, for sure. We’ve spoken a lot about SKA and how it’s going to be identifying things we hadn’t thought existed or, you know, hadn’t thought to look for, but it’s not the only big telescope coming up. Right. The Vera C. Rubin telescope and the LSST survey.
These are also working on it and there’s going to be some amazing stuff coming from that. So, yeah, it’s exciting times as always in astronomy and great that South Africa is involved in the LSST and getting more involved. It sounds like.
[00:41:47] Jacinta: Yeah, for sure. As we always say exciting things are coming up and tune in here to here to hear all about it.
[00:41:58] Dan: Alright, I think that’s it for today.
[00:42:00] Jacinta: Yeah. I think that about covers it.
[00:42:02] Dan: As always. Thanks very much for listening and we hope you’ll join us next time on The Cosmic Savannah.
[00:42:07] Jacinta: You can visit our website, thecosmicsavannah.com where we’ll have the transcript, links and other stuff related to today’s episode.
[00:42:15] Dan: You can follow us on Twitter, Facebook, and Instagram @cosmicsavannah that’s Savannah spelled S a v a n n a h.
[00:42:24] Jacinta: Special thanks today to Dr. Michelle Lochner for speaking with us.
[00:42:27] Dan: Thanks to our social media manager Sumari Hattingh.
[00:42:30] Jacinta: Also to Mark Allnut for music production, Jacob Fine for sound editing, Michal Lyzcek for photography, Carl Jones for astrophotography and Susie Caras for photographic design.
[00:42:40] Dan: We gratefully acknowledge support from the South African National Research Foundation and the South African Astronomical Observertory, as well as the University of Cape Town Astronomy Department.
[00:42:50] Jacinta: You can subscribe on Apple Podcasts, Spotify, or wherever you get your podcasts. And we’d really appreciate it, if you could rate and review us or recommend us to a friend.
[00:43:00] Dan: We’ll speak to you next time on The Cosmic Savannah.
I must say, my Netflix algorithm is shocking.
[00:43:08] Michelle: Yeah, it’s really bad. It really is bad. I’m trying to build a better one.
[00:43:14] Dan: Apparently Tick-tock is incredible. I wouldn’t know. I’m not on Tik Tok, but apparently its algorithm is amazing.
That’s a story for another day though.
[00:43:24] Jacinta: I don’t know. I think I’m just very predictable. Netflix gets me pretty much every time.
End of podcast:
365 Days of Astronomy
=====================
The 365 Days of Astronomy Podcast is produced by Planetary Science Institute. Audio post-production by Richard Drumm. Bandwidth donated by libsyn.com and wizzard media. You may reproduce and distribute this audio for non-commercial purposes.
This show is made possible thanks to the generous donations of people like you! Please consider supporting to our show on Patreon.com/365DaysofAstronomy and get access to bonus content.